Machine learning (ML) is steadily transforming how today’s organizations conduct their everyday operations. Corporate investment in machin...
Machine learning (ML) is steadily transforming how today’s organizations conduct their everyday operations. Corporate investment in machine learning and artificial intelligence is predicted to exceed $100 billion by 2025.
ML is enabling businesses to optimize their processes, grow their top-line, improve customer satisfaction and raise employee engagement. Here’s a look at some more specific ways ML is changing the way business owners think.
1. Personalized Customer Service
The potential payoff that can be realized from using machine learning to lower costs and improve customer service presents an exciting opportunity for entrepreneurs. By leveraging natural language processing, historical customer service data, and algorithms that can learn continuously from interactions with customers, businesses can build a customer service interface that delivers high-quality answers to questions asked.
The widespread adoption of chatbots is already demonstrating this can work. Human customer service agents need only step in when the chatbot is unable to conclusively respond to an inquiry.
2. Hiring
The most lucrative corporate jobs will usually pull in hundreds of resumes. It takes considerable time for recruiters to go through each of these resumes for every job opening advertised. This task is better handled by software that can quickly run through thousands of applications and identify the candidates with the credentials that are the best match for the role.
Such matching can be done by ordinary software but machine learning would take it to the next level. An ML-powered hiring app would go beyond a routine vetting of resumes and look past implicit biases such as flawed job descriptions. That way, they can still shortlist highly qualified candidates who do not exactly fit the specifications of the job description.
3. Finance
ML can speed up the exception handling process in many financial procedures. For instance, if a payment is received but lacks an order number, a finance department employee would ordinarily have to go through the outstanding orders to see which one the payment relates to.
ML software would reduce or eliminate this manual process by monitoring existing finance processes and gradually learning the responses needed in different situations. That way, the invoices can be automatically matched much faster thereby freeing staff to concentrate on more strategic work.
4. Brand Measurement
Automated applications can recognize logos, people, products and more. For example, advanced image recognition can track the position of logos in the video footage of a sports event.
That gives corporate sponsors a deep and detailed view of the return on their investment. The analyses would include specific metrics on the placement, duration, and quantity of corporate logos.
5. Detecting Fraud
The average business loses up to 5 percent of its revenues to fraud each year. By creating elaborate models based on social network information, historical transactions and external data sources, ML algorithms can use pattern recognition to identify outliers, exceptions, and anomalies.
This ensures the detection and prevention of fraudulent payments in real time including fraud that’s conducted in a way not known or seen before. For example, banks can use ML tools to pick up suspicious account activity while tax authorities can link the transaction trails of individuals with overlapping connections.
6. Predictive Maintenance
Traditionally, maintenance was either a reactive or proactive process. Machine learning is making it possible to have predictive maintenance. Unlike proactive maintenance where parts replacement and equipment servicing follows a strict schedule, predictive maintenance uses a more intelligent need-based approach.
For example, instead of waiting until a train is due for maintenance to perform the necessary servicing, ML software can detect unusual changes in the performance of certain parts so repairs can be done before the part fails.
7. Improved Supply Chains
ML enables the analysis of logistics data in context in order to predict and counter supply chain threats. ML algorithms can scan news feeds and social data in diverse languages to, for example, pick up news reports of a fire in a remote factory that supplies vital ball bearings necessary for car transmission.
8. Career Planning
ML-powered career recommendations could guide employees toward selecting paths that result in high levels of satisfaction, retention, and performance.
If an entry-level staff with a marketing degree hopes to be the head of the department someday, what type of work experience, education and in-house training should they obtain? In what order should they obtain these credentials that are relevant to each job level on their way to the top?
9. Drone-Based Asset Management
Drones run by ML software and equipped with cameras can regularly inspect the external surface of commercial structures such as airplanes, bridges, dams, silos and nuclear power plants. The ML application would then automatically analyze the photos to detect changes to the structure’s surface or any new cracks then send alerts to maintenance teams accordingly.
10. Retail Shelf Analysis
A sports drink company can utilize the power of ML to see whether its product displays are at the promised location in the retail store, that shelves are well and correctly stocked with the product, and that product labels are clearly visible to shoppers.
ML enables businesses to reimagine processes by wielding the power of digital intelligence. The potential for ML is enormous and is the reason technology vendors are heavily investing in artificial intelligence.
There are certainly formidable barriers such as the availability of substantial volumes of high-quality data that can be fed into ML algorithms. In some organizations, such data isn’t held in one place so part of the preparation before deploying ML would be to consolidate all useful data.
ML’s rise is inevitable. It’s not a matter of whether businesses will have to embrace it but rather which businesses will reap the benefits first by adopting ML the earliest.
ML is enabling businesses to optimize their processes, grow their top-line, improve customer satisfaction and raise employee engagement. Here’s a look at some more specific ways ML is changing the way business owners think.

1. Personalized Customer Service
The potential payoff that can be realized from using machine learning to lower costs and improve customer service presents an exciting opportunity for entrepreneurs. By leveraging natural language processing, historical customer service data, and algorithms that can learn continuously from interactions with customers, businesses can build a customer service interface that delivers high-quality answers to questions asked.
The widespread adoption of chatbots is already demonstrating this can work. Human customer service agents need only step in when the chatbot is unable to conclusively respond to an inquiry.
2. Hiring
The most lucrative corporate jobs will usually pull in hundreds of resumes. It takes considerable time for recruiters to go through each of these resumes for every job opening advertised. This task is better handled by software that can quickly run through thousands of applications and identify the candidates with the credentials that are the best match for the role.
Such matching can be done by ordinary software but machine learning would take it to the next level. An ML-powered hiring app would go beyond a routine vetting of resumes and look past implicit biases such as flawed job descriptions. That way, they can still shortlist highly qualified candidates who do not exactly fit the specifications of the job description.
3. Finance
ML can speed up the exception handling process in many financial procedures. For instance, if a payment is received but lacks an order number, a finance department employee would ordinarily have to go through the outstanding orders to see which one the payment relates to.
ML software would reduce or eliminate this manual process by monitoring existing finance processes and gradually learning the responses needed in different situations. That way, the invoices can be automatically matched much faster thereby freeing staff to concentrate on more strategic work.
4. Brand Measurement
Automated applications can recognize logos, people, products and more. For example, advanced image recognition can track the position of logos in the video footage of a sports event.
That gives corporate sponsors a deep and detailed view of the return on their investment. The analyses would include specific metrics on the placement, duration, and quantity of corporate logos.
5. Detecting Fraud
The average business loses up to 5 percent of its revenues to fraud each year. By creating elaborate models based on social network information, historical transactions and external data sources, ML algorithms can use pattern recognition to identify outliers, exceptions, and anomalies.
This ensures the detection and prevention of fraudulent payments in real time including fraud that’s conducted in a way not known or seen before. For example, banks can use ML tools to pick up suspicious account activity while tax authorities can link the transaction trails of individuals with overlapping connections.
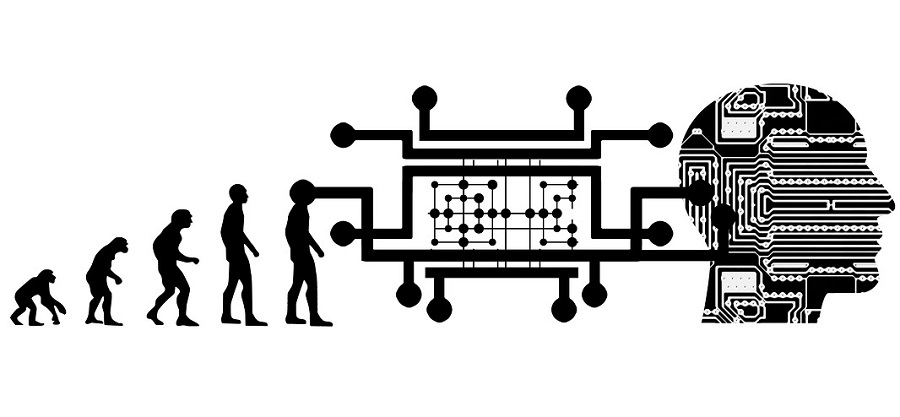
6. Predictive Maintenance
Traditionally, maintenance was either a reactive or proactive process. Machine learning is making it possible to have predictive maintenance. Unlike proactive maintenance where parts replacement and equipment servicing follows a strict schedule, predictive maintenance uses a more intelligent need-based approach.
For example, instead of waiting until a train is due for maintenance to perform the necessary servicing, ML software can detect unusual changes in the performance of certain parts so repairs can be done before the part fails.
7. Improved Supply Chains
ML enables the analysis of logistics data in context in order to predict and counter supply chain threats. ML algorithms can scan news feeds and social data in diverse languages to, for example, pick up news reports of a fire in a remote factory that supplies vital ball bearings necessary for car transmission.
8. Career Planning
ML-powered career recommendations could guide employees toward selecting paths that result in high levels of satisfaction, retention, and performance.
If an entry-level staff with a marketing degree hopes to be the head of the department someday, what type of work experience, education and in-house training should they obtain? In what order should they obtain these credentials that are relevant to each job level on their way to the top?
9. Drone-Based Asset Management
Drones run by ML software and equipped with cameras can regularly inspect the external surface of commercial structures such as airplanes, bridges, dams, silos and nuclear power plants. The ML application would then automatically analyze the photos to detect changes to the structure’s surface or any new cracks then send alerts to maintenance teams accordingly.
10. Retail Shelf Analysis
A sports drink company can utilize the power of ML to see whether its product displays are at the promised location in the retail store, that shelves are well and correctly stocked with the product, and that product labels are clearly visible to shoppers.
ML enables businesses to reimagine processes by wielding the power of digital intelligence. The potential for ML is enormous and is the reason technology vendors are heavily investing in artificial intelligence.
There are certainly formidable barriers such as the availability of substantial volumes of high-quality data that can be fed into ML algorithms. In some organizations, such data isn’t held in one place so part of the preparation before deploying ML would be to consolidate all useful data.
ML’s rise is inevitable. It’s not a matter of whether businesses will have to embrace it but rather which businesses will reap the benefits first by adopting ML the earliest.
COMMENTS